“The most reliable way to predict the future is to create it.” – Abraham Lincoln
Who would have thought that an 1860s' statesman would have sage advice for digital business leaders in 2020. Data, and the use thereof, is the hottest technology trend going right now. The recent Snowflake and Palantir IPOs are just the latest examples of market expectations for data-based applications. As a technologist, I understand why there is so much excitement. But as a service provider, I worry that the practical application of data and use of AI continue to be abstract and elusive for most of us. However, recent thinking in AI combined with Lincoln’s advice provide a way for all of us to catch this wave.
Amazon recently announced new applications for its Alexa feature known as “hunches.” For consumer facing companies like Amazon and Netflix, “hunches” are predictions of customer behavior. Alexa gets a hunch that a customer wants to lock their front door when over time it sees how the homeowner locks the doors, turns off the lights and sets the alarm by the bed. Initially Amazon and Netflix made “suggestions” based upon their “hunches” about what else you might like to buy or see; they took the safe route and left you in control. But now, Amazon is giving users the option of trusting Alexa to act upon its hunches; Alexa predicts the desired state for the home and acts to make it happen. It seems obvious, but simple steps based on good data can lead to important outcomes like a safe home.
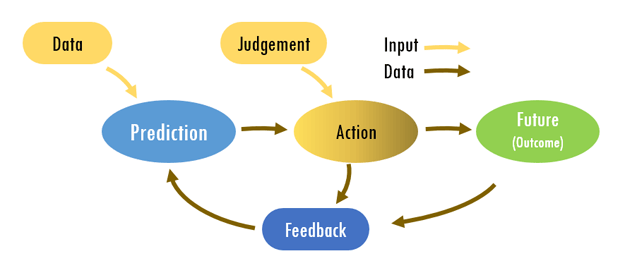
Figure 1: Anatomy of a Task defines a “prediction machine” – Agrawal, et. al.
https://sloanreview.mit.edu/article/what-to-expect-from-artificial-intelligence/
I like the construct of “hunches” in digital system designs because prediction provides a practical way to understand how to engage AI. I first came to this understanding via Dr. Ajay Agrawal’s book Prediction Machines. Prediction machines, as defined by Agrawal et. al., provide two key insights into the application of AI. First, their “Anatomy of a Task” diagram (Figure 1) informs the architecture and process for building a data-driven control loop that learns and drives to a desired outcome. The core of the machine is identifying the prediction that informs actions needed to reach the desired outcome. Alexa has a prediction machine that predicts the state the homeowner wants for their home at any given time and then takes actions that create that state.
Equipment finance, by its nature – providing funds today with the expectation of future repayment – has many prediction problems. Consider the residual value on a leased asset contract. Typically, equipment finance companies rely on “blue book” type models that forecast asset values as a function of time and condition to price the lease transaction. But once the money is released, the lessor moves to a “wait-and-see” model with knowledge of passing time but not equipment condition. A prediction-view of this problem would be: “How do I predict the value of the asset over the period of the lease contract?” Since the value of an asset is dependent upon its use and care during its lifetime, one could make a prediction if one had equipment use and care data. Today, that data exists. The “residual value prediction machine” would monitor the use, abuse and maintenance of the equipment to continuously forecast whether the value of the asset is at or above the contracted residual value (Figure 2).
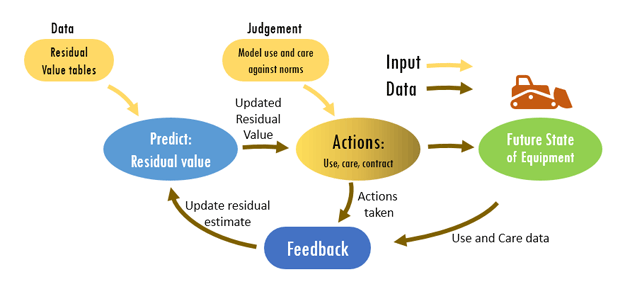
Figure 2: Prediction machine for residual value.
At this point, we see the second value of the prediction machine: It provides the opportunity to affect outcomes. When one automates a prediction machine, i.e. closes the loop, the machine continuously takes actions to reach an outcome – learning along the way which actions work best. Prediction machines not only learn from their mistakes, “I guess you didn’t want to watch Clint Eastwood in “The Bridges of Madison County,” they help create the future by acting on accurate hunches – “Here’s a fun Clint Eastwood film “Kelly’s Heroes.” Cha-ching!
I recently discussed the application of prediction machines in finance with Justin Bjerkaas, Founder and CEO of the Micah-Group. Not surprisingly, Justin was very familiar with Agrawal’s Prediction Machines. The Micah-Group is combining AI tools with their experience in banking to help finance organizations improve customer relationships and provide better experiences through data. They do this in three steps applying their AI tool “Micah.”
- They break down data-silos within institutions to aggregate and make available the mass of data available.
- Micah continuously “cleans” the data – removes out-of-date or contradictory data – while learning which data the decision maker trusts.
- Micah learns and automates the lower work – the simple workflow decisions, while providing better information faster for the higher work – the cognitive decisions that must be made by humans.
Micah predicts and learns how to help users make better decisions faster with data. Justin’s vision for Micah is not to replace the human, but to make humans more productive by learning which information helps them most – a 1+1 = 3 model for AI use.
"Innovation is an effect in economy and society, a change in the behavior of customers, of teacher, of farmers, of doctors, of people in general." – Peter Drucker – Innovation and Entrepreneurship
The value of a technology is often judged by the innovation it enables. The expectations for AI to learn and automate our complex world are high – nearly out of reach. Ironically, the key to applying AI and leveraging the plethora of data already available is to simplify the challenge by finding the prediction problem that enables better outcomes. When we view our challenges as prediction problems, we innovate by changing our problem-solving behavior. If we then deploy our prediction machines, we can automate behavior change of those we serve to provide better outcomes for both them and us. After all, higher residual values lead to lower lease payments. Lower lease payments lead to more deals.
Lincoln was close.
“The most reliable way to create the future you want is to predict it.”
Readers are encouraged to read parts 1 and 2 of this series, “The Optics of Data”:
Part 1: “Be Prepared” in a Digital World – How to Look Like You Already Knew This Was Coming!
Part 2: Want More and Better Data? Build Trust